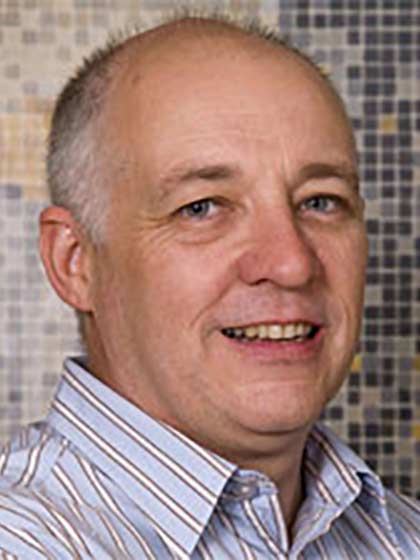
October 2021
Upcoming events by this speaker:
Now-Data Fabric, Mesh, and a Lakehouse
The first time I heard the words data warehouse was in 1985. A new and inexperienced systems architect, I was a member of a tiny team in IBM Ireland’s internal software laboratory. We had been asked to propose a new approach to providing consistent, reliable management information to sales and finance teams in IBM Europe who were struggling as the business underwent significant change in the types and range of hardware and software products being sold.
The technology solution was pre-set as the then emerging relational databases and would showcase IBM’s DB2 that had launched in 1983. Although relational databases would conquer the world by the mid-90s, at the time, they couldn’t match the power and function of the then dominant hierarchical databases, such as IMS, in operational systems. DB2 was looking for a market niche, and decision support was chosen.
We delivered an architecture for internal use in 1986 and went on to describe it publicly in 1988[1]. Among other components, the system contained a business data warehouse (BDW) structured around high-level business entities and a business data directory (BDD), both implemented in the relational model. Within the year, multiple large, international customers had contacted us and revealed they were attempting similar systems.
IBM launched the concept commercially in 1991 as “Information Warehouse,” but it was never a big success, and it was left to evangelists like Bill Inmon and Ralph Kimball, and software companies such as Teradata to popularise the concept and build the market.
Plus ça change, plus c’est la même chose
The reason for this short trip down memory lane is that it offers relevant architectural lessons today, when technological change is as extensive as it was in the 1980s. With the recent introduction of at least three new concepts, architectural considerations become increasingly important in choosing which, if any, of the data fabric, data mesh, or data lakehouse you should pursue.
These days, I caution clients that product selection should occur only after the conceptual and logical architecture have been well settled and a review of existing systems completed. However, our industry often takes the opposite path, with infrastructure software developers and vendors at the forefront of designing system architectures, driven by the “latest and greatest” software advances.
The data lake concept and, more recently, the data lakehouse, both coming from the open-source, extended Hadoop ecosystem, are good examples of this. The data lake has been hamstrung for years by a limited understanding of or adherence to data management and governance principles by many of its proponents, leading to well-documented data swamps. Data lakehouse discussions also centre largely on the technology features and possibilities offered by the underpinning products.
Technology does, of course, matter deeply. As in the 1980s, when we were seeing major shifts in data management from hierarchical/network databases to relational and in storage from central mainframes to distributed minicomputers and PCs, we are now in the midst of another sea change, with cloud computing, enormous data volumes, and artificial intelligence changing the technology landscape. Data fabric and data mesh have both emerged from this shift.
Gartner began talking about data fabric as far back as 2018. An outgrowth of their earlier thinking on logical data warehouse since 2012, data fabric is one of the Gartner Top 10 Data and Analytics Trends, 2021[2]. The underlying thinking is to improve data accessibility in a highly distributed, hybrid on-premises multi-cloud environment using a set of architectural and design principles around reusable data services, active metadata, semantic graphs, and AI.
In contrast, data mesh[3], an approach developed by ThoughtWorks’ consulting group in collaboration with several clients, resets thinking about the traditional and multiple stores of data (warehouses, marts, operational systems, etc.) created and used by the enterprise. A data-as-a-product mindset is proposed, supported by a domain-driven, distributed architecture and infrastructure as a platform. While this aligns well with microservices thinking and agile methods, the (near-)complete disavowal of consolidated and reconciled data stores to support cross-functional data use is worrying. Furthermore, the companies that have been most supportive of the approach—Netflix, Intuit, and Zalando—display data environments and organisations are probably considerably less complex than more traditional brick-and-mortar firms, particularly in financial services.
A Neutral Basis for Comparison
These three novel contenders—fabric, mesh, and lakehouse—show startlingly different approaches to the opportunities and challenges of this new era in technology and digital business. Comparing them with one another and, indeed, with existing solutions—such as data warehouse, lake, and logical data warehouse—cannot be easily undertaken with simple checkbox and scoring methods. Yet, a comparison is precisely what we must do to know if their strengths are of value or their weaknesses a problem in any specific enterprise, and if they offer enough “bang for your buck” to consider replacing existing systems, with all the work and risk that entails.
What we need is a neutral architecture, at both conceptual and logical levels, against which to measure up all the contenders, both old and new. Conceptual in order to understand the interplay of business needs and technological possibilities (and roadblocks). Logical for functional analysis and comparison of the different approaches. The architectural frameworks outlined in my 2013 book, Business unIntelligence[4], provide such a foundation. Taken together, they form the Digital Information Systems Architecture (DISA), which enables the required comparison.
To take one example, the information reliance/usage axis defines different scopes of reliance that apply to different information/data sets. Enterprise scope describes the core of a data warehouse; local scope, in contrast, applies to data marts; personal scope to spreadsheets. Enterprise level decisions should not be taken based on a spreadsheet—obvious, although often ignored in practice. Applying these same classes to a data mesh, built around data-as-a-product exposes challenges in delivering cross-enterprise reconciliation in a data mesh.
Conclusion
The original 1980s data warehouse architecture work was undertaken in a rapidly changing IT world with an eye to novel technology but with feet firmly on the ground of existing systems and well-defined business drivers. The 2020s requires a similar combination of approaches to deliver a set of solutions as long-lived and well-respected as data warehousing.
[1] Devlin, B.A. and Murphy, P.T., “An Architecture for a Business and Information System”, 1988, IBM Systems Journal, 27(1), bit.ly/EBIS88
[2] Gartner Top 10 Data and Analytics Trends, 2021, gtnr.it/3fLaK2l
[3] Dehghani, Z., “How to Move Beyond a Monolithic Data Lake to a Distributed Data Mesh”, 2019, bit.ly/3dtLrl3
[4] Devlin, B., Business unIntelligence, 2013, bit.ly/BunI-TP2